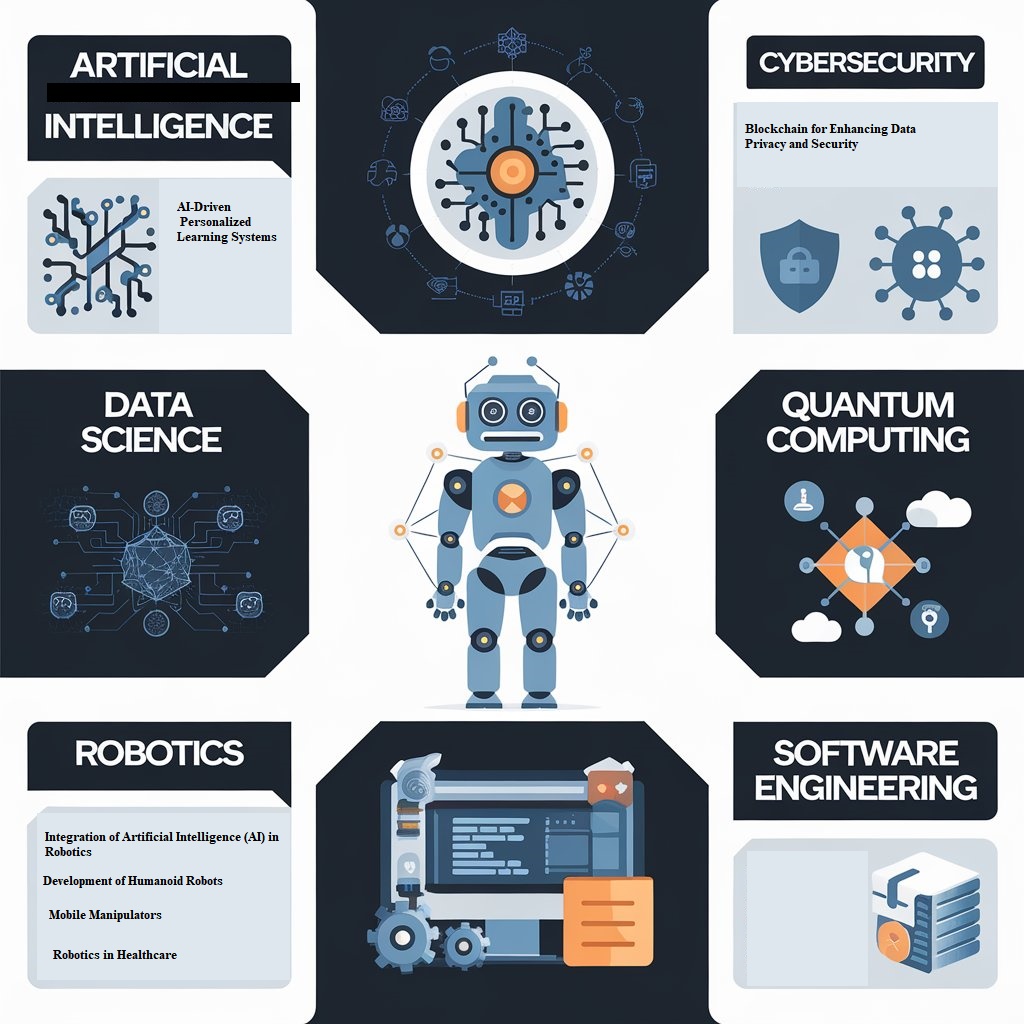
Computer science is a rapidly evolving field driven by technological advancements and innovative research. Emerging artificial intelligence (AI), machine learning, cybersecurity, and blockchain trends reshape industries and influence how we interact with technology. As digital transformation accelerates, researchers and students explore solutions to real-world challenges, from improving cybersecurity frameworks to advancing AI-driven automation.
Recent breakthroughs highlight the growing importance of these fields. For instance, AI is revolutionizing healthcare with predictive diagnostics, while post-quantum cryptography is being developed to counter the threats posed by quantum computing. Similarly, blockchain technology is integrated into cybersecurity protocols to enhance data integrity and prevent cyberattacks.
We will explore key research areas in computer science backed by recent studies and emerging trends. Understanding these topics is essential whether you are a student searching for research ideas or a professional keeping up with technological advancements.
What makes a strong computer science research topic
Relevance
A strong computer science research topic must be relevant to current technological trends and societal needs. Relevance ensures the research is timely and valuable, addressing pressing challenges or exploring cutting-edge innovations like artificial intelligence, quantum computing, cybersecurity, or data privacy. Topics tied to real-world applications, such as AI in healthcare, blockchain in finance, or machine learning for climate prediction, are more impactful because they solve actual problems that affect people or industries. For instance, a topic like “Developing Explainable AI for Medical Diagnosis Systems” is both relevant and timely, as it addresses the growing integration of AI in healthcare and the critical need for transparency in its decisions.
Originality and Novelty
Originality is the heartbeat of meaningful research. A good topic should introduce a fresh perspective, explore a new research gap, or apply known technologies in a unique context. Rather than rehashing well-trodden problems, original research seeks out unexplored questions or under-researched domains. For example, using federated learning in rural healthcare environments or applying blockchain in environmental conservation can uncover valuable insights that haven’t been deeply studied. To ensure novelty, it’s helpful to examine the latest conference papers and journal articles to find what’s missing in the current body of knowledge.
Clear Research Problem or Question
A strong research topic must present a well-defined research question. It should be clear about what is being studied, why the research is important, and what outcome is expected. Vague or overly broad topics can become unmanageable and dilute the research impact. Instead, a focused question like “How can federated learning models be made robust against adversarial attacks in decentralized environments?” provides a clear direction for investigation. This clarity guides methodology, scope, and analysis, making the research process smoother and more productive.
Feasibility
No matter how exciting a topic may sound, it must be doable within your available time, skills, and resources. Feasibility includes access to the required datasets, tools, or experimental platforms and whether the research can realistically be conducted given technical or institutional constraints. For instance, a small thesis project may not have the capacity to build a fully functional AI assistant but could analyze sentiment classification techniques using publicly available datasets. A manageable topic like “Performance Comparison of Two Sorting Algorithms on Large Datasets” could be a wise choice if your resources are limited but you want to explore algorithm efficiency.
Potential for Contribution
A strong research topic should contribute to both academic knowledge and real-world practice. This could involve proposing a new algorithm, improving an existing system, or solving a practical problem with an innovative solution. Academic contributions might include theoretical frameworks or novel methodologies, while practical contributions might result in usable software tools, enhanced security protocols, or improved data analysis techniques. For example, researching “Designing a Secure IoT Architecture for Smart Homes” can produce insights valuable to both academia and the tech industry, especially in today’s interconnected digital environments.
Ethical and Responsible
With the increasing influence of computer science in everyday life, ethics cannot be overlooked. A research topic must consider the ethical implications of data use, algorithmic fairness, and security. This includes ensuring user privacy, reducing bias, obtaining consent, and building systems that are secure and inclusive. For example, a topic like “Bias Detection in Hiring Algorithms Using Intersectional Fairness Metrics” directly confronts ethical issues in AI and offers pathways for creating more just and equitable systems. Responsible research promotes trust in technology and safeguards against harm.
Trending Computer science Research ideas
1. Quantum Computing Advancements
-
Quantum Error Correction and Scalability: Companies like Amazon and Google are making significant strides in quantum computing. Amazon’s introduction of the ‘Ocelot’ chip represents a breakthrough in error correction and scalability, addressing key challenges in the field. Similarly, Google’s ‘Willow’ chip has demonstrated extraordinary computational speed, capable of performing tasks in minutes that would take conventional computers an astronomical amount of time.
-
Financial Applications of Quantum Computing: JPMorgan Chase has achieved a milestone by demonstrating a real-world application of quantum computing in generating certified random numbers, crucial for cryptographic security and data protection. This positions the bank at the forefront of financial institutions exploring quantum technology.
2. Artificial Intelligence Interpretability and Ethics
-
Understanding AI Decision-Making: Researchers are delving into the internal mechanisms of AI models to enhance transparency and trust. Experiments, such as those conducted with AI assistants like Claude, aim to decipher how AI knowledge is represented and processed, which is vital for ensuring ethical and reliable AI applications.
-
AI in Scientific Discovery: The integration of AI in scientific research is accelerating discoveries across various domains. Microsoft’s AI for Science lab emphasizes AI’s potential to revolutionize fields like chemistry, physics, and biology by expediting complex research processes.
3. Thermodynamic Computing
-
Harnessing Thermodynamic Fluctuations: Innovations in thermodynamic computing propose utilizing inherent thermodynamic fluctuations for computation, offering an alternative to traditional and quantum computing paradigms. Startups like Extropic are developing chips based on this principle, potentially leading to more efficient computing systems.
4. AI in Education
5. Graph Neural Networks (GNNs)
Latest Computer Science Topics
1. Artificial Intelligence & Machine Learning
- Explainable AI: Making neural networks more interpretable.
- AI for medical diagnosis and healthcare.
- Bias mitigation techniques in AI models.
- Energy-efficient deep learning models.
- Reinforcement learning in autonomous robotics.
- AI-driven cybersecurity threat detection.
- Emotion recognition using deep learning.
- AI-powered fake news detection.
- Machine learning for personalized education.
- Neural networks for financial market prediction.
2. Data Science & Big Data
- Scalable algorithms for real-time big data analytics.
- Privacy-preserving data mining techniques.
- Data-driven fraud detection models.
- Predictive analytics for climate change impacts.
- Human behavior modeling using big data.
- AI-based traffic flow optimization.
- Sentiment analysis of social media data.
- Anomaly detection in sensor networks.
- AI for personalized marketing strategies.
- Blockchain integration in big data security.
3. Cybersecurity
- AI-based intrusion detection systems.
- Quantum cryptography techniques.
- Privacy-preserving computation using homomorphic encryption.
- Deepfake detection methods.
- Secure communication protocols for IoT.
- Zero-trust architecture in cybersecurity.
- Cyber threat intelligence using machine learning.
- Secure multi-party computation protocols.
- AI in malware detection and classification.
- Ethical hacking: Automated penetration testing tools.
4. Blockchain & Cryptography
- Blockchain for secure voting systems.
- Smart contracts for supply chain transparency.
- Privacy-focused blockchain protocols.
- AI-driven fraud detection in blockchain networks.
- Blockchain for healthcare record management.
- Decentralized identity verification systems.
- Cryptographic algorithms for post-quantum security.
- Blockchain-based digital rights management.
- Secure cryptocurrency wallet design.
- Energy-efficient blockchain consensus algorithms.
5. Internet of Things (IoT)
- AI-powered IoT security solutions.
- Smart city applications using IoT.
- Edge computing in IoT environments.
- Blockchain-based authentication in IoT devices.
- Privacy-aware IoT data sharing frameworks.
- AI-driven predictive maintenance for IoT devices.
- IoT-based smart home automation.
- Secure firmware updates for IoT devices.
- Swarm intelligence in IoT networks.
- Digital twins for real-time IoT monitoring.
6. Cloud Computing & Edge Computing
- AI-powered cloud resource management.
- Serverless computing security challenges.
- Energy-efficient cloud data centers.
- AI-driven cloud anomaly detection.
- Edge computing in healthcare applications.
- Cloud-based disaster recovery solutions.
- Privacy-preserving AI models on the cloud.
- Secure data migration strategies in cloud computing.
- Federated learning in cloud-based AI models.
- Decentralized cloud storage security techniques.
7. Computer Vision
- AI for real-time object detection in autonomous vehicles.
- Gesture recognition for human-computer interaction.
- AI-powered document image analysis.
- Deep learning for satellite image analysis.
- Real-time crowd density estimation using surveillance cameras.
- AI for visual speech recognition.
- Image forgery detection using deep learning.
- AI-driven wildlife monitoring using drones.
- Vision-based emotion recognition in video calls.
- AI-powered sign language translation.
8. Human-Computer Interaction (HCI)
- Brain-computer interface for assistive technology.
- AI-driven chatbots for mental health support.
- Smart wearables for real-time health monitoring.
- Virtual reality (VR) for education and training.
- AI-driven adaptive UI/UX personalization.
- Haptic feedback in VR environments.
- Voice recognition improvements using AI.
- AI-powered automatic summarization of long texts.
- AI-driven accessibility tools for visually impaired users.
- Intelligent virtual assistants for workplace productivity.
9. Robotics & Automation
- AI-powered swarm robotics.
- Robot-assisted surgery advancements.
- Reinforcement learning for autonomous drones.
- AI-based navigation in self-driving cars.
- AI-powered disaster response robots.
- AI-driven warehouse automation.
- Humanoid robots for customer service.
- AI-powered agricultural robots.
- Autonomous drone-based delivery systems.
- AI-based gesture-controlled robotic arms.
10. Software Engineering & Development
- AI-assisted software bug detection.
- AI-driven automated code refactoring.
- AI-based test case generation for software testing.
- Blockchain-based software licensing.
- Low-code/no-code AI-powered development.
- AI-driven software project estimation models.
- AI-based automated UI testing frameworks.
- AI-powered code generation tools.
- Predictive analytics for software maintenance.
- AI-driven DevOps automation.
Summary Checklist for a Strong Topic
Conclusion
As technology continues to evolve, research in computer science plays a crucial role in shaping the future. Advancements in AI, cybersecurity, quantum computing, and blockchain are not just theoretical concepts but are actively transforming industries and daily life. Keeping up with the latest trends allows students, professionals, and researchers to contribute to solving pressing technological challenges and driving innovation.
To stay informed, exploring reputable research sources such as IEEE Xplore, ACM Digital Library, and arXiv can provide valuable insights into cutting-edge developments. Whether you aim to contribute to AI-driven automation, enhance digital security, or explore the vast potential of quantum computing, staying engaged with emerging research is key to thriving in this dynamic field.
Read more on Best Computer Science Assignment Help